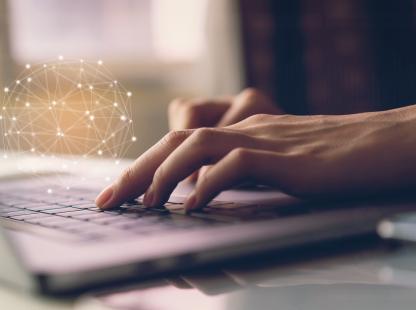
Course Description
Our courses provide a world-class, practice-based, data-driven education that translates immediately into expertise you can take to the workplace.
Each academic course bears 3 credits, taught over a 15-week semester at a single weekly session of 3 hours (typically 6pm - 9pm).
- Math 661 - Applied Statistics
- HRM 601 - Organization Behavior
- IS 601 - Web Systems Development
- IS 657 - Spatio-Temporal Urban Analytics
- IS 665 - Data Analytics for Information Systems
- IS 677 - Information Systems Principles
- IS 684 - Business Process Innovation
- IS 688 - Web Mining
- CS 506 - Foundations of Computer Science
- CS 602 - Java Programming
- CS 608 - Cryptography and Security
- CS 610 - Data Structures and Algorithms
- CS 630 - Operating Systems Design
- CS 631 - Data Management Systems Design
- CS 632 - Advanced Database Systems Design
- CS 635 - Computer Programming Languages
- CS 636 - Data Analytics with R Programming
- CS 634 - Data Mining
- CS 643 - Cloud Computing
- CS 644 - Introduction to Big Data
- CS 645 - Security and Privacy in Computer Systems
- CS 656 - Internet and Higher Layer Protocols
- CS 670 - Artificial Intelligence
- DS 650 - Data Visualization and Interpretation
- CS 673 - Software Design and Production Methodology
- CS/DS 675 - Machine Learning
- DS 677 - Deep Learning
Math 661 - Applied Statistics (Andrew Pole)
Extracting information from data is the application of statistical description, analysis, and inference. Businesses, industry, academia, governments face these questions every day, looking for opportunities and seeking solutions for emergent problems: How much bandwidth can that streaming compression technology free up? Will we gain customers if we improve the audio quality? How should we examine performance data from four different designs of a prosthetic limb? What is the reliability of different component manufacturing processes? How do we allocate funding to maximize relief impact ? In this course you will learn basic and advanced mathematical and statistical tools and techniques for extracting information from your data, big or small, and to make quantitatively justified statements about general populations of concern (customers, patients, supply chain choke points, …). You will also use real-world data from many settings in the current leading data analytic environments R and Python to learn how to address these business, research, and social needs.
HRM 601 - Organization Behavior (Julie Ancis)
This course is essential to learning how to facilitate and manage collaboration among diverse teams as you investigate and solve real-world problems. This course focuses on organizational structures and functioning. We will learn how personality, attitudes, and values affect workplace behavior and the impact of leadership style on decision-making. Learn how events and conditions, including technological advances, affect the functioning of an organization. Analyze the role of groups in organizations and increase the probability that people will work well in teams. Tackle real business problems through real-world case studies and applied learning opportunities. Develop self-awareness and awareness of others through personal assessments.
IS 601 - Web Systems Development (Ryan Tolboom)
This course trains in the development of web-based systems using Python and the Django web framework. You will learn to program with Python and Javascript, currently two of the three most in-demand programming languages, and learn to develop a web based system through an intensive, hands-on project that requires application of real-world problem-solving skills. You will also learn to utilize the Django web framework, one of the ten most popular web frameworks and currently in use by Instagram and Pinterest. Model-View-Template design, the Django templating language, and object relational mapping for database access will be covered in this course. At the conclusion of the course, you should be able to create a bespoke web interface for a standard internal business application.
DS 650 - Data Visualization and Interpretation (Aritra Dasgupta)
“We’re not just fighting an epidemic; we’re fighting an infodemic” - The WHO has observed that we are currently facing unprecedented challenges due to data-driven misinformation about the ongoing pandemic which can have severe consequences. The key contributors to this “infodemic” are often poorly designed visual charts, which can disinform or misinform, and do so, at scale. The need to learn the basics of visualization design and interpretation, both as a data consumer and a data practitioner, is more relevant and consequential now than ever before. Visualization techniques, when designed effectively, have emerged as a game-changer in data science, business Intelligence, and journalism domains for communicating data-driven insights. In this course you will learn the theory and application of visual design and how to reason about what makes a visualization good or bad. You will learn how to employ principles imbibed from computer science, human perception, cognition and information design to make your charts error-free, effective, and actionable. You will learn why visualization is needed in the data science pipeline, how it can be used to solve real-world problems, and how to distinguish between “good”, "bad", or “misleading” visualizations. You will gain hands-on experience in building interactive visual interfaces and dashboards using Python, Tableau and Javascript.
IS 657 - Spatio-Temporal Urban Analytics (Hua Wei)
This is a project-based course in which we will focus on visualization of high-dimensional data collected about urban populations. Students will have an option of working on an individual project or a group project where they will focus on visual communication of data-driven insights. To get the most out of this course, it is necessary that students have taken the IS 650 course and are familiar with basic data visualization techniques.
Students will get to choose from a number of project options and the project goal can be tailored based on a match between their preferences and the course objectives. They will work closely with the instructor and his team of PhD students and the schedule (of meetings/deliverables) can be mutually agreed upon before the start of the course.
Students will be evaluated based on the progress they make towards meeting the project deliverables. The focus will not solely be on a final product but on the lessons they learn while committing and rectifying mistakes along the way.
The project options include, but are not limited to, the following topics: data discovery, where the goal is to semantically integrate openly available spatio-temporal data (e.g., NYC open data) for driving decision-making about various facets, like health, education, economy, etc., of an urban population; educational data mining, where openly available college and university datasets (across states and multiple years) will be combined with students’ economic and demographic data for identifying potential bias and lack fairness in admissions’ criteria; studying information consumption, where social media data (e.g., Twitter) combined with sentiment analysis can be used to analyze the nature of content that propagates through social networks in times of a global pandemic/”infodemic” and reflect on the need for each person be their own moderator for preserving their mental health.
All of the aforementioned projects comprise an element of research. They require students to exercise their critical thinking skills and will have ample opportunity for them to come up with innovative approaches/solutions, and in turn, contribute directly to the research projects.
IS 665 - Data Analytics for Information Systems (Lin Lin)
This graduate level course introduces students to the world of data analytics from an information systems perspective, focusing on the application of various data analysis techniques in business practices. We cover a wide spectrum of topics ranging from fundamental statistics to database, data warehouse, data visualization, and data mining. Being an introductory course, our approach is “shallow and wide”, emphasizing on giving students a complete view of the data analytics profession, covering as many different sub-areas as time allows while not diving too deep into any one specific domain. The goal is to serve as a “guided tour” for students to gain knowledge about the different sub-areas of data analytics and understanding of which area is a best fit for their personal developments. More in-depth materials and discussion for each sub-area will be provided upon students’ requests. Course topics include the rudiments of probability and random variables, data visualization, data warehousing and OLAP analysis, dashboard, scorecard, data mining algorithms, optimization techniques, DSS and knowledge systems.
IS 677 - Information Systems Principles (Yi-Fang Wu)
This course introduces the field of Information Systems; the study of how people and organizations should use information technologies effectively. We examine the major areas in the field, analyzing the major issues, trends and problems. We survey the role of information systems in organizations and how these systems support organizational objectives and organizational structure, as well as provide competitive business advantages. We discuss basic concepts such as the systems point of view, the organization of a system, the nature of information and information flows, as well as how people process information and related cognitive concepts. We also examine various types of information system applications such as big data, cloud computing, e-commerce, supply chain, decision support, and enterprise systems.
IS 684 - Business Process Innovation (David Ullman)
This course adopts a balanced approach to business process innovation (BPI) that includes both incremental improvement and re-engineering. It specifically examines the concept of a service-oriented architecture (SOA) and the use of web services as a way to enable scalable and adaptive business processes. Students will learn how to develop process maps using the Business Process Modeling Notation (BPMN) and design process improvements to achieve efficiency, effectiveness, compliance and agility objectives. The focus of the course is on ways in which information technology can be used to manage, transform and improve business processes.
IS 688 - Web Mining (Hai Phan)
Web mining aims to discover useful information and knowledge from the Web hyperlink structure, page contents, and usage logs. It has direct applications in e-commerce, Web analytics, information retrieval/filtering, personalization, and recommender systems. Employees knowledgeable about Web mining techniques and their applications are highly sought by major Web companies such as Google, Amazon, Yahoo, MSN, and others who need to understand user behavior and utilize discovered patterns from terabytes of user profile data to design more intelligent applications. The primary focus of this course is on Web usage mining and its applications to business intelligence and biomedical domains. We learn techniques from machine learning, data mining, text mining, and databases to extract useful knowledge from the Web and other unstructured/semistructured, hypertextual, distributed information repositories. This data could be used for site management, automatic personalization, recommendation, and user profiling. Topics covered include crawling, indexing, ranking, and filtering algorithms using text and link analysis, applications to search, classification, tracking, monitoring, and Web intelligence. Programming assignments give hands-on experience. A group project highlights class topics.
CS 506 - Foundations of Computer Science (Jing Li)
This course provides the mathematical and analytical foundations of computer science and its applications to various areas in CS. The course covers the material traditionally known as “discrete mathematics”, with special emphasis on CS applications and analysis of algorithms. Discrete Mathematics is viewed as the pillar or language of CS. One needs to be fluent in it to work in many CS fields including data science, machine learning, and software engineering. The course topics include sets and logic, proof techniques, proof by induction, functions and relations, analysis of algorithms, recursion, recurrence equations, divide-and-conquer design technique, counting methods (permutations and combinations), basic discrete probability, and if time permits, introduction to number theory and a brief introduction to graphs and trees.
CS 602 - Java Programming
CS 608 - Cryptography and Security (Fuad Hamidli)
This course involves computational methods providing secure Internet communication. Among the topics covered are: Security threats in communication systems; conventional cryptography: substitution and transposition codes; distribution of secret key over the Internet; principles of public-key cryptography; RSA and other public-key cryptographic methods; and digital signature protocol.
CS 610 - Data Structures and Algorithms (Ioannis Koutis)
From data organization and retrieval, to social networks and mapping solutions, algorithms have revolutionized software, which in turn has changed the economy and our lives. This course introduces you to a core set of ideas and techniques that underly a multitude of existing algorithms, and are commonly used as 'building blocks' in the design of new algorithms. You will also gain exposure to issues of computational efficiency that are of paramount importance in the development of software products and time-critical applications. The course is centered around a hands-on project that helps you acquire practical experience with algorithms for network analysis. Emphasis is also given on boosting your computational problem-solving skills via algorithmic problems that appear in programming interviews by top companies in the tech and finance sectors.
CS 630 - Operating Systems Design (Jing Li)
Operating systems are the foundation of computer science and computer systems. A deep understanding of OS allows one to develop programs and applications that are efficient and scalable when running on the underlying hardware. This course prepares you with the knowledge of operating systems concepts and designs that appear in computer systems, via discussing the basic operating system abstractions, mechanisms, and their implementations. In particular, topics we will cover include operating system designs, multiprogramming, timesharing, resource scheduling, synchronization of concurrent processes, management of hierarchical storage, embedded and real-time systems, virtualization, and distributed systems.
CS 631 - Data Management Systems Design (Canan Eren)
A relational database organizes data into tables which can be linked—or related—based on data common between them, and you can communicate with these databases using the standard Structured Query Language (SQL). This course is about designing, creating and querying relational databases. As data becomes more and more important, we see an explosion of specialized databases (OLAP, time-series, document, graph, etc.), data processing tools (Hadoop, Spark, Flink), data buses (Kafka, RabbitMQ), etc. We also have more applications that rely on this data infrastructure, whether data visualization tools (Tableau, Grafana, PowerBI, Superset), web frameworks (Rails, Django) or custom-built data-driven applications, built on a complex stack, with infrastructure on the bottom and applications on top. To make this work, we need an interface that allows the different layers of this stack to communicate with one another. This can be done using SQL, and the course will teach you this technology and allow you to gain hands-on experience with SQL to design, create, and manipulate a relational database by developing a real-world application.
CS 632 - Advanced Database System Design (Canan Eren)
Covers the rapidly changing concepts and principles of modern database systems and database programming based on SQL. Additional topics may include: advanced data modeling, OODBs, parallel and distributed database systems, XML and NO-SQL databases, Web-database systems, active databases, multimedia and text databases, database security, query optimization, indexing techniques, concurrency control, system performance, and data warehaousing.
CS 634 - Data Mining (Pantelis Monogioudis)
The process of extracting signals from massive amounts of noisy data is a multi-step endeavor that involves, data analysis, visualization, learning algorithms, model analysis and verification. In this course you will learn not only what methods to use at each one of these stages but also, how to design and architect end-to-end pipelines that deliver on your technical and, ultimately, business objectives. Using real-world use cases from Zillow, Uber and other technology companies, you will use Python and cutting-edge open source machine learning libraries and tools to mine massive, publicly available repositories of structured (tabular) as well as unstructured (e.g. natural language) data. The skills acquired in this course are essential for aspiring data analysts or scientists across all data-driven industries.
CS 635 - Computer Programming Languages
DS 636 - Data Analytics with R Programming (Kumar Mani)
This course will teach how to program in R and how to use R for effective data analysis. Students will learn how to install and configure R necessary for an analytics programming environment and gain basic analytic skills via this high-level analytical language. The course covers fundamental knowledge in R programming. Popular R packages for data science will be introduced as working examples. It will familiarize you with the commonly used analytical techniques in data science and develop the way of data science thinking: learn how to preprocess, explore and interpret real data and learn how to model real problems using computational techniques. The format of the course will include lectures by the instructor, computing labs, class discussion, directed reading and student presentation or project.
CS 643 - Cloud Computing (Cristian Borcea)
The objective of the course is to introduce modern database systems. The course focuses on the following issues: conceptual data modes, the Entity-Relationship model, the Relational Model, formal and commercial Database Languages, the Relational Algebra, Database Design, Storage (File Organizations, External Hashing, Indexing), Query Processing and Optimization, Formal Database Design (Normalization) and Transaction Processing.
CS 644 - Introduction to Big Data (David Bader)
Data science is a rapidly emerging discipline at the intersection of machine learning, algorithms, and statistics. Solving real-world problems in domains such as financial services, cybersecurity, and health informatics requires the generation, storage, management, and analytics, on massive data sets. This course will take you on a journey of developing high performance data analytics for massive data sets using accelerated computer architectures, state-of-the-art software frameworks, and real-world case studies. In-depth topics will focus on mathematical modeling, scalable algorithms, and high-performance computing, for fast and practical solutions. Together we will explore real-world data modeling and prediction from the Data Science Design Manual and learn How 45 Successful Companies Used Big Data Analytics to Deliver Extraordinary Results.
CS 645 - Security and Privacy in Computer Systems (Fuad Hamidli)
Security has become pervasive in our daily use of computers and networks because of the increasing reliance of critical industries on computer systems and networks, the ever-growing software complexity and attack sophistication, and the need to ensure data security and privacy both in transit and when being stored. The course covers fundamental principles of building secure systems and techniques to ensure data security and privacy. Topics include authentication and access control mechanisms, physical security, operating and file system security, malicious code threats and software security, web security, database security, and recent security incidents. You will be exposed to the theoretical underpinnings of cybersecurity and also get a chance to apply these concepts in several projects.
CS 656 - Internet and Higher Layer Protocols (Kumar Mani)
This course introduces the protocols and standards of the TCP/IP suite that govern the functioning of the Internet. The material covered in class is a top-down approach on introduction, discussion, and analysis of protocols from the data-link layer to the application layer. Alternative protocols to the TCP/IP suite and new protocols adopted by this suite are discussed. Numerical examples related to network planning and proocol functioning are analyzed.
CS 670 - Artificial intelligence (Pantelis Monogioudis)
Fundamental concepts and general techniques in artificial intelligence. The main topics include goal tree search, logic and deduction, adduction, uncertainty, fuzzy logic, knowledge representations, machine learning, vision, and action planning. The LISP programming language is used extensively. Students are required to do programming assignments, complete a programming term project, and review case studies.
CS 673 - Software Design and Production Methodology
CS/DS 675 - Machine Learning (Ioannis Koutis)
Machine Learning develops computer programs that can improve their performance by tapping into existing data and taking feedback from the environment. Systems based on ML have already exceeded human performance in several tasks, including image medical image classification and games like Chess and Go. ML has also made leaps in even more complicated tasks, like Natural Language Processing or self-driving vehicles, and it has even produced art that imitates the style of human artists! This course offers an intense introduction to the fundamental ML concepts and algorithms that constitute the core of these spectacular developments. It takes you on a tour from the basic mathematical notions and algorithms to some of the recent developments, e.g. Deep Networks or Recurrent Networks. You will gain exposure to cutting-edge ML development tools such as Scikit-learn and TensorFlow via hands-on assignments and projects that instill a working and immediately applicable knowledge of ML methods and will prepare you for more advanced ML courses.
DS 677 - Deep Learning (Ioannis Koutis)
Deep Learning (DL) is a subfield of Machine Learning that has delivered disruptive technologies, created AI algorithms that outperform humans in various tasks, and paves the way for broader advances in Science. DL consists of a set of specialized techniques that exploit the abundant availability of data and computational power to build models that are composed of multiple processing layers and learn representations of data at multiple levels of abstraction. Only a few years back, the development of DL models required significant expertise, but the introduction of open source DL libraries like TensorFlow and PyTorch has opened the area to scientists and professionals with more diverse backgrounds. The course opens with a review of Artificial Neural Networks that guides you through TensorFlow (or PyTorch) and enables you to build novel ANN architectures. Then it presents the evolution of progressively deeper architectures for Convolutional Neural Networks, that addressed various training difficulties and led to very successful image classification models. In this spatial context, you will also learn about Generative Adversarial Networks that are behind the fascinating 'Deep Fake' images and videos. The course then takes you to the emerging applications of Recurrent Neural Networks in temporal data, including Natural Language Processing. You will also learn how Deep Learning combined forces with Reinforcement Learning to create models that can play games. The course will also touch upon selected topics like the ability of deep networks to generalize, techniques for 'pruning' deep networks in order to make them more computationally efficient, and successful applications of DL methods in the Sciences.